Read the paper in its full form at Towards Data Science.
—
A Framework for a Human-Centered AI Based on the Laws of Nature
Integrating natural and artificial intelligence
By Tom Kehler
Presented to the Boston Global Forum High-level Conference “AI Assistant Regulation Summit: Fostering a Tech Enlightenment Economy Alliance” at the Harvard Faculty Club. The paper presented here is an expansion of that talk.
We are at many crossroads. The one in sharp view in recent months is AI, resulting in a spectrum of responses from terror to glee. No doubt you have by now experienced the delight of playing with ChatGPT. Many have joined the rush to adoption. Others suggest this current expression of AI is yet another race to the bottom where we throw caution to the wind because we must. Everyone else is doing it, so we must do so as well. Aggregate bad behavior that no one wants — but exists because no one knows how to build trust is a shadow that comes with technological advances. Technology is not the enemy. Failure to collaborate and come together in trust leads to reckless adoption that could lead to harm.
In this brief overview, I hope to provide you with a framework for an AI future that builds trust and reduces risk.
That framework was first unveiled by the founders of science and the scientific method dating back to the Enlightenment. The scientific method that followed formed the foundation for building trusted knowledge — a collaborative process totally dependent on collective human intelligence and trust in the emergent elegance offered in nature.
We recommend employing the power of collective human intelligence and the intelligence built into the physics of living systems to guide us forward.
For nearly 70 years, the scientific pursuits of AI centered on building handcrafted models of the natural intelligence and cognitive skills of humans using the tools of symbolic representation and reasoning. They were capable of explaining how they solved a problem. Trust was built by observing their reasoning.
For the past 20 years, Statistical Learning from the explosion of data offered by the Internet yielded spectacular results — from self-driving cars to the Large Language Models that bring us together today. In particular, transformer deep learning architectures unlocked generative AI’s powerful potential, which has created the impressive results we see today.
The concern that brings us here today relates to three fundamental problems. For the first time in the history of information technology, we are not enforcing the concept of data provenance. Thus, these tremendous generative powers can be persuasive purveyors of misinformation and undermine trust in knowledge. The second concern is explainability — the systems are black boxes. The third concern is they need a sense of context.
These three points of weakness go crossways with the three pillars of the scientific method of citation, reproducibility, and contextualization of results. What do we do?
Judea Pearl says, ‘You are smarter than your data,’ We agree. The human capacity for counterfactual thinking is far more powerful than anything we can learn from correlative patterns in our past data.
Large Language Models and deep learning architectures in general develop models of intelligent behavior based on pattern recognition and correlation models from data. Generative output from LLMs employ human’s in the loop to filter and train the results. The risk remains however that generation of content containing misinformation may not be caught in the filtering process.

Five years ago in a MIT Technology Review interview, one of the fathers of deep learning, Yoshua Bengio, stated:
“I think we need to consider the hard challenges of AI and not be satisfied with short-term, incremental advances. I’m not saying I want to forget deep learning. On the contrary, I want to build on it. But we need to be able to extend it to do things like reasoning, learning causality, and exploring the world in order to learn and acquire information.”
It is extremely unlikely that current models based on correlation of patterns in historical data capture the complexity of the human brains abilities. The imaginative power of the human brain and its abilities to generate cause models based on experience must be engaged as an integral part of future AI models. We propose an approach that incorporates human collective intelligence and a model of the human brain.
Larry Page, Serge Bryn, and Terry Winograd found that citation indexing could lead to a scalable way to order information on the web. The PageRank algorithm brought order to the web. The mathematics of citation indexing brings order to understanding information sharing in human collaboration.
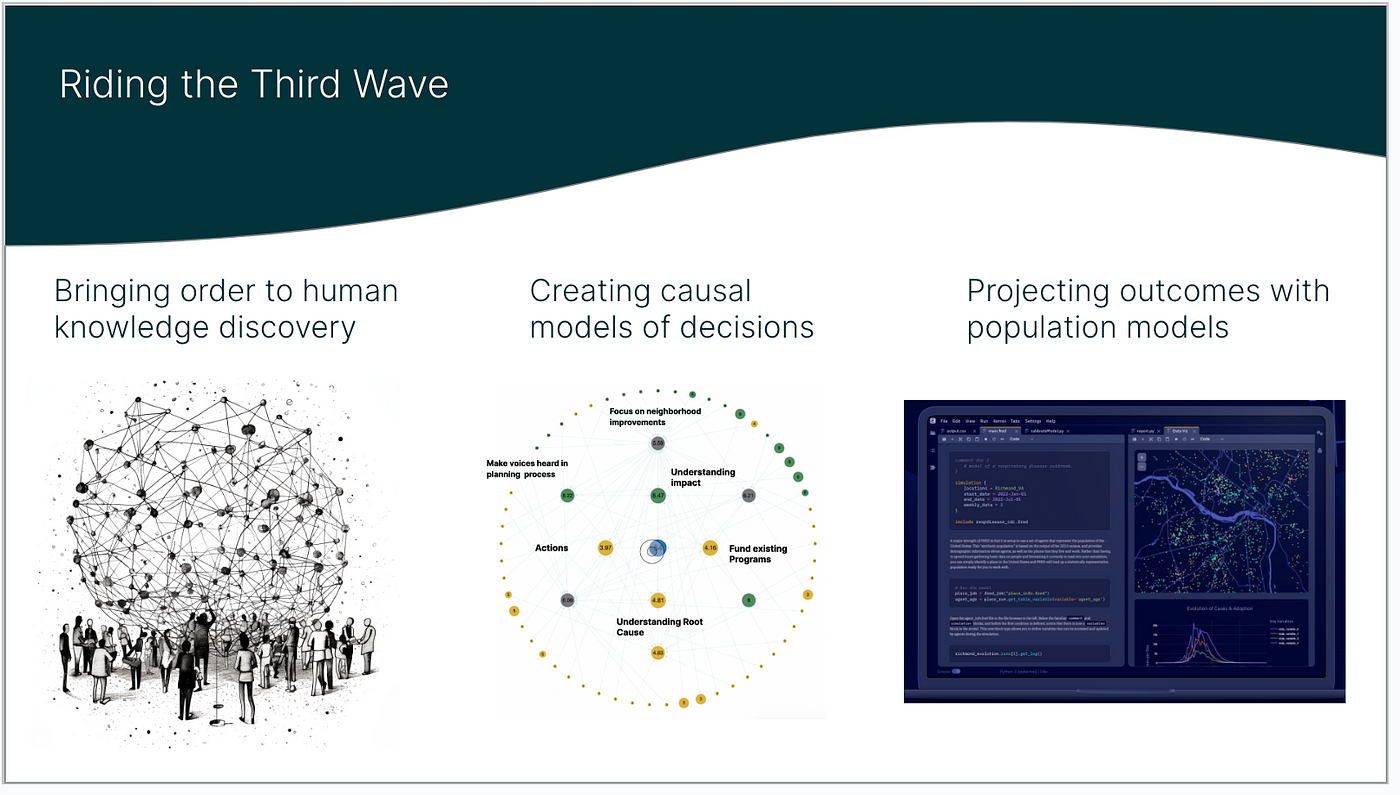
A next generation of AI that integrates human collective reasoning, developed in the past eight years, uses a citation indexing approach as a knowledge discovery process. It allows knowledge discovery at scale, supporting citation, reproducibility, and contextualization. We propose this as part of a framework going forward.
Collective reasoning seeks to learn a community or group’s aggregated preferences and beliefs about a forecasted outcome. Will a product launch create the results we want? If we change our work-from-home policy, will we increase or decrease productivity? What policy for using ChatGPT and LLMs will be best for our organization? All of these questions require learning a group’s ‘collective mind’ on the predicted outcome. The collective reasoning process employs AI technology to learn a model of the collective mind. The process is single-blind, reducing bias. The system was tested for four years on groups of 20 to 30 experts/investors predicting startup success, and they/it was >80% accurate). Those beliefs and predictions are mapped into collective knowledge models — Bayesian Belief Networks.
We can embed the critical elements of the scientific knowledge discovery process in how we co-create or collaborate to solve complex problems. Rather than have AI undermine trust in knowledge, we propose using AI to learn collective knowledge models, causal models that retain provenance, explainability, and context. This is a critical component of a new enlightenment — bringing the scientific method to collaboration.
Collective reasoning allows learning the intentions of a group. An agent-based simulation is useful in forecasting the impact of a proposed solution. Synthetic models of populations based on public data allow scaling and forecasting the impact of co-created solutions, and we propose that as part of the framework. One of the partner companies in this initiative has built a significant capability to simulate impact at scale, applying it to the social implications of disease propagation.
What about the foundation of AI going forward? What have we learned in 68 years since the summer of 1956 when AI was born? The first few decades developed the components that form the current AI landscape. The mathematics of cooperative phenomena and the physics of magnetism plays an exciting role in linking it all together. Hopfield, in 1982, demonstrated that the emergent collective computational capabilities of artificial neural networks mapped directly to the mathematical physics of spin glasses. The same mathematics of cooperative phenomena describes the emergence of order out of chaos as shown in the murmuration of starlings photo at the beginning of this article.
Recently, Lin, Rolnick, and Tegmark at MIT showed that the reason deep and cheap learning work so well is tied to the the laws of physics. Bayesian learning is reformulated as a fundamental method used in quantum and classical physics — The Hamiltonian. Explicitly focusing on the roots of AI in natural laws should be the focus of future AI development.
Central to it all is learning order out of disorder. A new wave of studies in the brain takes learning at the order/disorder boundary to a theory for creating living intelligence systems — the Free Energy Principle.
The FEP is a framework based on Bayesian learning. The brain is thought of as a Bayesian probability machine. If sensory inputs do not match expectations, a process of active inference seeks a way to minimize the uncertainty going forward. The difference between what we expect and what we sense is called surprisal and is represented as free energy (energy available for action). Finding a path with minimum free energy is equivalent to finding a path that reduces surprise (uncertainty).
An AI based on FEP adapts locally and scales based on variational free energy minimization principles used throughout the physical and biological sciences. Bioform Labs is building out a Biotic AI that adapts and learns. Unlike second-generation AI, which requires massive training data sets and complicated cost functions, AI based on the physics of living systems is adaptive and lives within an ecosystem. It can be designed to respect the states that lead to the needs of living systems.
Technology to get started on this new framework is applicable today. We don’t need to halt the development of AI. Collective reasoning applies to questions we need to ask ourselves about the impact of AI in a wide variety of specific contexts. How will AI affect investments in technology? How will it change our hiring practices? What impact will it have on our community?
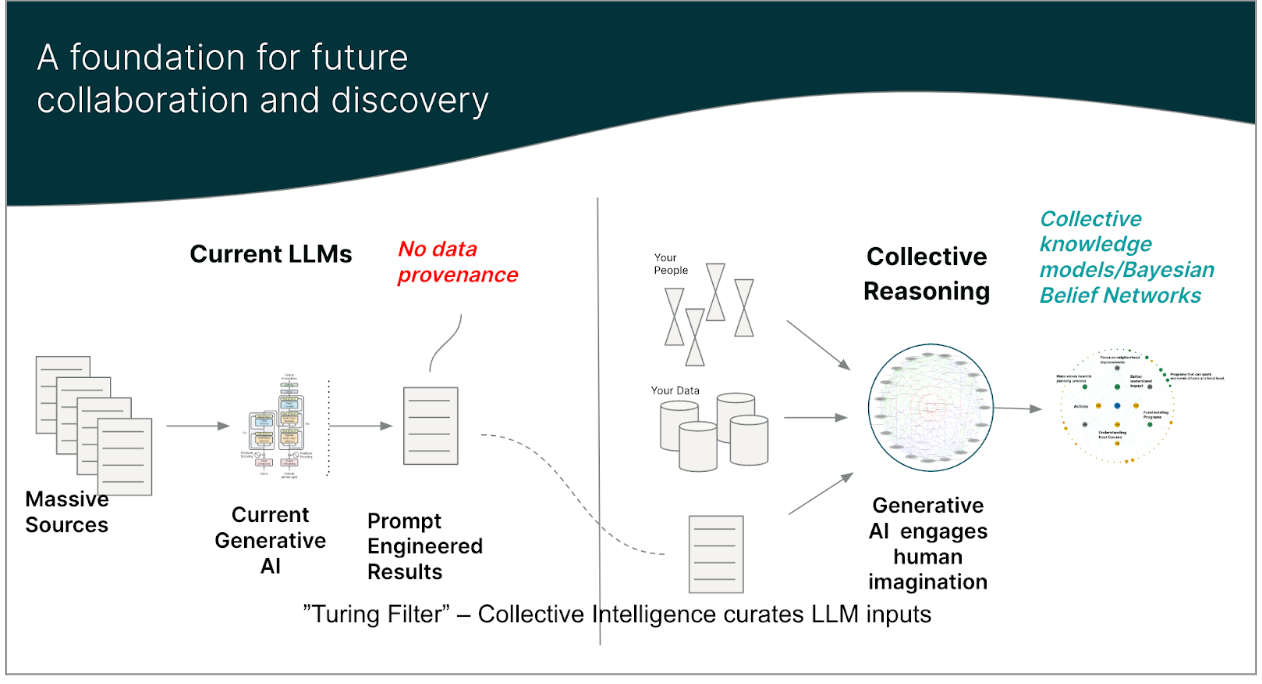
In addition, it is possible to engage ChatGPT and LLMs in ideation while retaining a privacy boundary. Ideas streamed in from an LLM can be curated and employed in specific private contexts. Curated and contextualized contributions are managed in a patented private LLM environment.
Collective reasoning learns intentions and possible solutions. Agent-based simulations forecast impact. We no longer need to think of organizations as rigid. New types of organizational governance, based on active inference, support adaptively learning a survival path forward. We believe this framework is a vision for the future that will
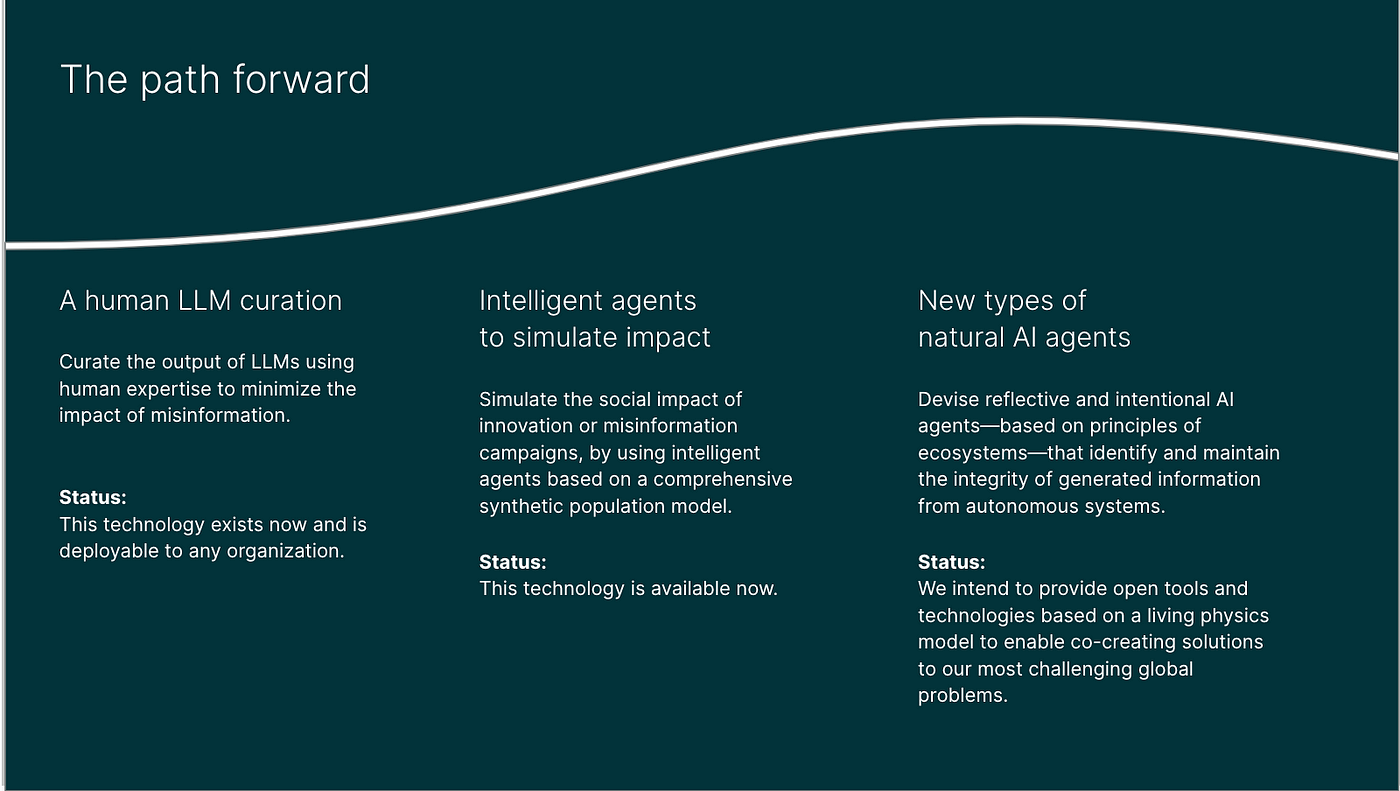
We can then set out to build a new AI-empowered Enlightenment that reconnects with collective human intelligence that led to the human progress we have enjoyed. As the Enlightenment freed science from the tyranny of religious authority, a new initiative, AI-empowered Enlightenment, provides a path to collaborate and co-create solutions — to free us from unintended consequences of the current wave of AI frenzy.
In conclusion, Large Language Models provide highly useful capabilities that are unfolding at an impressive rate. Read the warning labels! ChatGPT does warn not to implicitly trust the results but to use critical thinking. Don’t expose private data. For private data Figures 3 and 4 demonstrate a way to experiment by allowing ChatGPT or other ‘agents’ to provide inputs to a curated collaboration with human experts with results kept in a privately managed LLM context. This approach allows exploring the generative power of LLMs while retaining control of private intellectual property.
—
Read the paper in its full form at Towards Data Science.